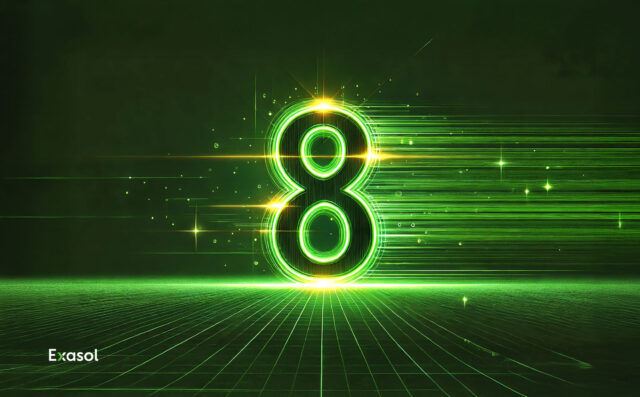
Blog
Exasol 8 Release 2025.1 is now GA: explore built-in AI, GPU support, faster performance, new Admin UI, and Snowflake/Databricks connectors.
Continue readingExplore how data sovereignty and AI are shaping enterprise strategy, with a focus on compliance, cloud repatriation, and building trusted infrastructure.
Continue readingIn today’s digital enterprise, data is everywhere—hidden within systems, processes, and transactions. Yet making sense of this data, especially when it comes to operational efficiency, remains a challenge. That’s where …
Continue readingExasol: The Fastest Analytics Engine on the Market When it comes to analytics databases, performance is the ultimate differentiator. Exasol has built the fastest analytics engine in the market, powered …
Continue readingIn today’s fast-paced business environment, your operational database needs to be agile—handling day-to-day transactions without the burden of historical data. That’s where the Jumpmind SymmetricDS connector for Exasol steps in. …
Continue readingHow to build sovereignty-driven strategies that strengthen trust, improve data security, and future-proof your business in a fast-changing digital economy.
Continue readingThis article explores 2025’s key data sovereignty trends, and why repatriating data is gaining momentum.
Continue readingDiscover what drives cloud based server cost. Compare pricing models from Exasol, Snowflake & BigQuery to find the most predictable, scalable solution.
Continue readingStep-by-step guide to building a secure, on-prem Text to SQL solution using local LLMs, dynamic prompt engineering, and Exasol schema metadata.
Continue readingDiscover why enterprises are shifting from cloud to on-prem, driven by data sovereignty, rising costs, and the need for control and compliance.
Continue reading