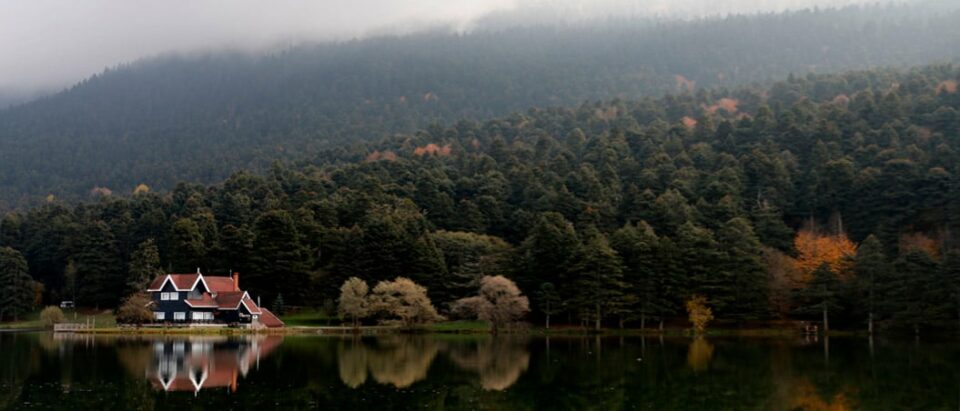
Data Lake vs. Data Warehouse vs. Data Lakehouse
The data lake versus data warehouse debate has raged for over a decade. But is a resolution finally in sight with the so-called ‘data lakehouse’, or is this just another example of a new buzzword generating hype? Exasol’s Market Intelligence Lead, Helena Schwenk, investigates.
Why are we debating data warehouse versus data lake yet again?
This question has been asked for years by data professionals like data scientists or data engineers, as the merits of one approach have been weighed up against the other. The debate, however, has grown ever more heated in recent years, thanks to the greater prevalence of the cloud-based solutions for data analytics workloads, the brittleness of Hadoop deployments and the emergence of a new concept: the data ‘lakehouse’.
If that phrase is new to you, you’re not alone – it’s a fairly new concept. But in short, the data lakehouse refers to a hybrid data architecture that aims to mix the best of a data warehouse and data lake.
Data lake or data warehouse? Similarities, differences and overlaps
To understand how a data lake, data warehouse or data lakehouse can underpin a modern analytics infrastructure, it’s worth unpicking some of their similarities and key differences. In terms of similarities of a data lake and a data warehouse, all are fundamentally used for the management of transactional and operational data that form the basis of business intelligence and advanced analytical workloads. This means they’re used for a wide-ranging set of analytical use cases across both business and developer functions. While a data lake is used for scenarios where you want to store and explore vast ammounts of raw data, a data warehouse is ideal for business intelligence, reporting or traditional data warehousing tasks.
However, it’s also worth remembering that each, data lake and data warehouse, serve different goals as borne out by their definitions. For example, a data lake and a data warehouse are two distinct approaches to data storage and management, each with its own set of characteristics and use cases, and they are each ideally suited to a particular data structure.
Data Warehouse
A Data warehouse is optimized for well-known, predefined and repeatable analytics needs that can be scaled across many users in the organization. As such, data warehouses are best suited to more structured data and governed data – such as in the financial services or healthcare sectors – and often involve a complex ETL process to transform and use a rigid schema-on-write approachad. Data warehouses are suited to SQL-based queries, high levels of concurrent access and high-performance data access requirements.
Data Lake
A data lake stores data in its raw, unprocessed form, which allows organizations to collect and store raw data without worrying about the format in which it will be used. Data lakes can handle structured data, semi-structured data and unstructured data captured from a diverse array of data sources. Data lakes are also likely to contain semi-structured or unstructured data types that aren’t subject to rigorous governance. Data lakes are highly scalable and are therefore suitable for Big Data applications. They support a range of different processing styles and approaches, including machine learning and batch-orientated workloads. Data lakes aren’t typically optimized for performance and the demands of production delivery – such as concurrency, latency and workload management. Data lakes have a flexible schema-on-read approach.
Some overlaps between a data lake and a data warehouse do occur. For instance, a data warehouse can also be used for operationalizing data science whereby machine learning models are run against governed data. And a data lake can introduce data analysis using approaches that leverage star schemas for batch orientated queries, for example.
Data Lakehouse
On the other hand, a data lakehouses aim to combine elements of data warehousing with core elements of the data lake. Put simply, they are designed to provide the lower costs of cloud storage even for large amounts of raw data alongside support for certain analytics concepts – such as SQL access to curated and structured data tables stored in relational databases, or support for large scale processing of Big Data analytics or machine learning workloads.
While this sounds good in practice, the lakehouse is an emerging and immature concept – which means there are differing views on how best to realize it. The main reason for this is that there are proponents on either side of the architectural divide. Those on the data warehouse side of the fence build the lakehouse around relational technology concepts, while those on the data lake side have roots in machine learning and Spark processing where support for processing Java, Python and R workloads is paramount.
Although the lakehouse is an interesting concept, it’s still very ill-defined and (unsurprisingly) subject to a lot of hype and speculation. And though a heated discussion will continue for some time, it’s unlikely to irrevocably remove the need for either the data lake or data warehouse, and more importantly, overturn the enormous amount of innovation in the market.
This is especially true when you consider the decades of data warehouse development seen in areas such as query and performance optimization, in-database analytics, columnar storage and compression.
The strategy for data democratization is co-existence
Choosing between a data warehouse or data lake or, dare I say, lakehouse need not be an either/or decision. You’re unlikely to find that replacing a data lake with a data warehouse is an optimum solution. Instead, it’s about recognizing the similarities and key differences between data lakes, data warehouses and data lakehouses and using each architectural design for its strengths, or even combining their uses.
There is often a lot of redundancy across data stores in an organization, so having the ability to store and process large volumes of data across both a data lake and warehouse can help bring some order to the chaos. It’ll also enable businesses to scale their analytics projects more effectively and less time-consuming and help democratise data within their organizations. This approach of co-existence of data lakes and data warehouses draws on the key differences seen as strengths of each architectural design to serve a wider number of use cases than neither a data lake nor a data warehouse can support independently.
Another good example of this co-existence is when the insights generated in a data lake (or lakehouse) are propagated into a data warehouse to be consumed by a wider audience in a repeatable and scalable manner. Moreover, the need to access multiple analytical data stores in distributed locations can also be supported through data virtualization, enabling data to be federated across the data lake, lakehouse and the data warehouse.
Delivering a modern data infrastructure requires flexibility
These examples illustrate the need for a more flexible approach, enabling analytics use cases that are well-defined and repeatable (via the data warehouses) alongside support for instances that are more experimental, machine learning- and developer-led (via the data lake). Likewise, both – data lakes as well as data warehouses – can support different tasks and user roles across the spectrum; from non-technical and business-oriented, from cross-company to department-specific to data scientist, data engineer and developer centric.
Trying to address the constantly changing data environment with all its various data sources is a tall order, and many data professionals like data scientists are consumed with trying to overcome the roadblocks to data access and availability. Making sure the data warehouse and data lake work together and not against each other is part of the process.
It’s likely too that the promise of the lakehouse offering a one-size-fits-all (but largely unproven) approach will initially be a step too far the many who’ve invested significantly in data lakes and data warehouses. But this kind of architectural conversation is important to have. The future is data-driven and data analytics and the architectures that underpin it will no doubt evolve in the coming years, so we’d love to hear your thoughts on how the discussion “data lake or data warehouse (or lakehouse)” will take shape.
Data Lake, data warehouse (or lakehouse)? Let us know what you think on our social channels – it’s going to be fascinating to see how the debate – and wider market – evolves next.
Data Lake vs. Data Warehouse vs. Data Lakehouse: Overview
Data Lake | Data Warehouse | Data Lakehouse | |
---|---|---|---|
Type of Data | Best for semi-structured and unstructured data | Best for structured data | Best for structured, semi-structured, and unstructured data |
Purpose | Best for artificial intelligence task and machine learning | Best for business intelligence (BI) and data analytics | Best for research, flexible storage, machine learning, and data analytics |
Cost | Storage is cost-effective, flexible, and fast | Storage can be costly and time-consuming | Storage is cost-effective, flexible, and fast |
It’s likely too that the promise of the lakehouse offering a one-size-fits-all (but largely unproven) approach will initially be a step too far for the many who’ve invested significantly in data lakes and data warehouses. But this kind of architectural conversation is important to have. The future of data analytics and the architectures that underpin it will no doubt evolve in the coming years, so if you are ready to transform your organization’s data strategy and unlock the full potential of your data today. Exasol’s high-performance in-memory analytics database offers lightning-fast query response times and unmatched scalability.